Machine Learning x Protein Engineering Proposal
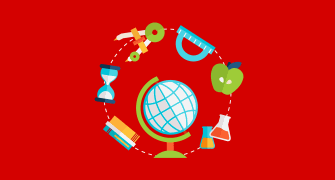
Machine Learning x Protein Engineering Insights
Welcome to the Machine Learning x Protein Engineering proposal quiz! This interactive quiz aims to gather insights and innovative ideas on how machine learning can advance protein engineering.
- Define key problem statements
- Explore differentiating factors
- Identify potential areas of interest
- Share your expertise and contribute to the proposal
Please select your name.
Adrian Matthew Mak
Ang Ee Lui
Ann Koay
Chandra Verma
Chen Xixian
Derek Smith
Giulia Rancati
Kumar Selvarajoo
Marco Klaehn
Michael Sullivan
Nic Lindley
Sebastian Maurer-Stroh
Shawn Hoon
Tan Teck Leong
Wong Han Teng
Wong Fong Tian
Thomas Lautier
What will be the differentiating factor for this ML X PE proposal? What has not been covered by PIPS and ACDP?
Following last week's discussion, which of the following areas continue to be of interest to you? For additional suggestions, please list them at the end of the survey (Question 13).
Swapping domains as a parameter for Machine Learning (beyond single point mutation)
Predicting non-naturally occurring catalysts/substrates/compounds/pathways
Extrapolate learning beyond training data
ML X Gene Annotation (benchmark: DeepRibo, DeepEC, UniRep; Splice AI, SpliceFinder)
ML X Retrosynthesis Pathway Design (benchmark: RetroPath 2.0; SMARTS; Apply ML principles from chemical retrosynthesis to enzymatic reactions; Semisupervised Gaussian Process for Automated Enzyme Search)
ML X Fermentation Process Optimisation (benchmark: DoE; Oyetunde et al. PLoS One. 15 Jan 2019)
ML X Transcription-Metabolic Network Regulation (includes: improve Transcription Factor-DNA binding; identification of key parameters; prevent lost flux and overcome bottlenecks)
What are the constraints preventing exploration of areas you have selected in Question 4? (Examples: de novo predictions; "missing" information from external databases as input; "noise"/variability among different conditions; single common output for various reaction conditions; predictions are specific to one type of organism/organ/strain/catalyst, etc.)
Give examples of new and/or improved products/processes that can be expected from this proposal? (Examples: novel/improved ML methodology; creation of new ML platform; ML-driven novel enzyme production using synthetic biology, etc.)
Please nominate a suitable lead for this proposal.
Adrian Matthew Mak
Ang Ee Lui
Chen Xixian
Derek Smith
Kumar Selvarajoo
Marco Klaehn
Michael Sullivan
Tan Teck Leong
{"name":"Machine Learning x Protein Engineering Proposal", "url":"https://www.supersurvey.com/QPREVIEW","txt":"Welcome to the Machine Learning x Protein Engineering proposal quiz! This interactive quiz aims to gather insights and innovative ideas on how machine learning can advance protein engineering.Define key problem statementsExplore differentiating factorsIdentify potential areas of interestShare your expertise and contribute to the proposal","img":"https:/images/course8.png"}
More Quizzes
Platform Quiz
639
Single cell sequencing and spatial transcriptomics
5224
Is it a tool or application ???
8418
RNA Isolation Quiz 2 - Concepts
14711
Quiz 2
5220
L9: Why the Future does not need us?
8413
Data Advent Quiz
420
Quiz of adlik & LF AI & Data
52111
Data Quiz. How much do you know about Big Data?
10573
Frazer Lab CARDiPS Election . . . DEADLINE to vote: Monday 10/10/16 @ Noon
630
Cas9 Crisper thoughts
520
Facial Recognition Training
1059