Depi
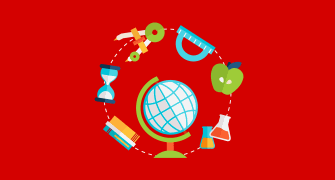
Stochastic Processes and Probability Quiz
Test your knowledge on stochastic processes and probability theory with our comprehensive quiz. With 56 challenging questions, this quiz covers a range of topics including random variables, autocorrelation, and independence.
- Understand the intricacies of stochastic signals.
- Evaluate your grasp of probability density fun
ctions. - Explore the relationships between random variables.
May two mutually exclusive events be independent too?
Always.
Never.
Only when one of them is the certain event
Only when one of them is the impossible event.
Only when one of them includes the other one.
Only when the two events are complementary.
Only when events are uncorrelated.
Only when events are correlated.
May the functiun q(ω) = 20 sinc(20ω) be the power spectral density
Yes, because the function is even.
Yes, because the function is even and positive.
Yes, because the function is even, real-valued and positive
No, because the function may take negative values.
No, because the function is odd.
No, because the function does not admit an inverse Fourier transform.
No, because the function is odd, and may take negative values.
Yes, because the function is the Fourier transform of an even function.
What can be said on the joint probability density function (PDF) of a pair of independent random variables that have, each, a normal distribution of order one?
The joint PDF is never normal.
The joint PDF is not always normal.
The joint PDF is normal only if the variables are uncorrelated.
The joint PDF is normal only if the variables are correlated.
The joint PDF is always normal.
The joint PDF is normal only if the variables have zero mean.
The joint PDF is normal only if the variablesDFs of order one are identical.
Nothing can be said of the joint PDF.
What can be said of the stationarity of a stochastic signal which is ergodic in the sense of the mean?
The signal may by stationary
The signal may is wide-sense stationary, not strict-sense stationary.
The signal may is strict-sense stationary, not wide-sense stationary.
The signal is wide-sense stationary.
If the signal were ergodic in the sense of autocorrelation, then it would be stationary.
The signal is not stationary.
The signal is stationary only in the sense of the mean, not in the sense of autocorrelation.
Nothing can be said on signal ̧s stationarity.
The correlation between independent random variables ξ and η that have, each, a uniform distribution over [0, 1] is:
0
1
0.5
0.25
0.75
0.2
0.6
0.8
The correlation between random variables ξ and η that have a joint normal distribution, with parameters m1 = 4, m2 = 2, σ1 = 4, σ2 = 3, ϝ = −0, 5 is:
1
-1
-2
2
-3
3
6
0
If the correlation between ξ and η is 0, then:
Sunt independente
Sunt decorelate
Nu sunt independente.
Nu sunt decorelate
Nu se poate spune nimic despre gradul lor de corelat ̧ie.
Sunt independente, dar corelate
Sunt decorelate, dar nu se poate spune nimic despre gradul lor de dependent ̧ ̆a
Sunt ̧si independente, ̧si decorelate
Poate funct ̧ia Rξ(τ ) = sinc(20πτ ) cos(2π104τ ) fi funct ̧ia de autocorelat ̧ie a unui semnal aleator stat ̧ionar?
Nu, ˆıntrucˆat nu este maxim ̆a ˆın origine
Nu, ˆıntrucˆat nu este par ̆a.
Nu, ˆıntrucˆat ia valori negativ
Nu, ˆıntrucˆat valoarea funct ̧iei la infinit este negativ ̆a
Da
Nu, ˆıntrucˆat transformata Fourier a funct ̧iei nu este par ̆a.
Nu, ˆıntrucˆat transformata Fourier a funct ̧iei ia valori negative
Nu ˆıntrucˆat transformata Fourier a funct ̧iei are valori complexe
Este semnalul avand functia de autocovariatie Kξ(τ ) = 10−|τ| 10 daca |x| ≤ 10 0 ın test ergodic ın sensul mediei?
Nu, intrucat functia nu respecta teorema ergodicitatii mediei
Da, caci functia este para
Da, caci functia, avand suport finit, respecta teorema ergodicitatii mediei
Nu se poate preciza cu sigurant ̧a.
Da, c ̆aci semnalul este stat ̧ionar, ̧si deci, implicit ergodic ˆın sensul mediei
Nu, ˆıntrucˆat media semnalului depinde de timp
Da, c ̆aci valoarea la infinit a funct ̧iei tinde la 0
Da, c ̆aci funct ̧ia este par ̆a ̧si pozitiv ̆a
If autocorrelation functions of both input and output signals of a linear, time-invariant system are known, can the system’s point-spread function be determined?
Da, ˆıntotdeauna
Numai ˆın situat ̧ia ˆın care ̧stim ̧si intercorelat ̧ia dintre cele dou ̆a semnale
Nu ˆıntotdeauna, c ̆aci densitatea spectral ̆a de putere a semnalului de ie ̧sire poate avea zerouri
Numai ˆın cazul ˆın care funct ̧ia de transfer a sistemului are valori pozitive
Nu, ˆıntrucˆat nu putem calcula ˆıntotdeauna densit ̆at ̧ile spectrale de putere ale celor dou ̆a semnale
Nu ˆıntotdeauna, c ̆aci densitatea spectral ̆a de putere a semnalului de intrare poate avea zerouri
Nu, ˆıntrucˆat avem nevoie ̧si de mediile celor dou ̆a semnale
Nu, ˆıntrucˆat nu putem calcula faza funct ̧iei de transfer
What can be said of two random variables, knowing that the mean of their product equals the product of their means?
Sunt independente, dar nu se poate spune nimic despre corelat ̧ia dintre ele
Nu se poate spune nimic, media produsului este egal ̆a cu produsul mediilor ˆıntotdeaun ̆a
Sunt dependente, dar decorelate
Sunt decorelate, dar nu se poate spune nimic despre dependent ̧a lor
Sunt independente, deci, decorelate
Nu se poate spune nimic, pentru a se evalua corelarea este nevoie de valoarea covariat ̧iei
Sunt corelate, deci nu pot fi independente
Sunt independente, pentru calculul coeficientului de corelat ̧ie avem nevoie ̧si de dispersiile celor dou ̆a variabile
What can be said of events A and B with P(A ∩ B) = P(A)?
If A occurs then B occurs
If B occurs then A occurs
A and B cannot occur simultaneoulsy
A and B are independent
A and B occur simultaneously.
A and B are complementary
P(A|B) = P(A)
B is the certain event
May function R(τ ) = K if |τ | < T0 0 otherwise be the autocorrelation function of a stochastic signal?
Yes, because it is even
Yes, because it is even and it reaches its peak in the origin
Yes, because it meets all properties of an autocorrelation function
No, as, even though it meets all properties of an autocorrelation function, the power spectral density may take negative values
No, as it does not meet the condition of the mean ergodicity theorem
No, as it does not admit the Fourier transform.
Nu, as the signal mean cannot be computed
Yes, as it is the inverse Fourier transform of an even function
What can be said on the joint probability density function (PDF) of a pair of independent random variables that have, each, a normal distribution of order one?
The joint PDF is never normal
The joint PDF is not always normal
The joint PDF is normal only if the variables are uncorrelated
The joint PDF is normal only if the variables are correlated
The joint PDF is always normal
The joint PDF is normal only if the variables have zero mean
The joint PDF is normal only if the variablesDFs of order one are identical
Nothing can be said of the joint PDF
What can be said of the stationarity of a stochastic signal which is ergodic in the sense of the mean?
The signal may by stationary
The signal may is wide-sense stationary, not strict-sense stationary
The signal may is strict-sense stationary, not wide-sense stationary
The signal is wide-sense stationary
If the signal were ergodic in the sense of autocorrelation, then it would be stationary
The signal is not stationary
The signal is stationary only in the sense of the mean, not in the sense of autocorrelation
Nothing can be said on signal¸s stationarity
What is the significance of a discontinuity in the cumulative distribution function of a random variable?
The random variable is discrete
The probability density function of the random variable will be discontinuous too
The random variable cannot take the value where the discontinuity occurs
The probability density function of the random variable will contain a train of Dirac impulses.
The random variable is continuous
The random variable will have non–zero probability to take the value where the discontinuity occurs.
The random variable is mixed.
The probability that the random variable takes values in a small interval around the discontinuity is zero.
ξ and η are Gaussian, independent, random variables with ξ = 0, η = 5, σξ = 4 and ση = 3. The value of the coefficient r of the joint probability density function of ξ and η is
0
1
2
3
May function Rξ(τ ) = sinc(20πτ ) cos(2π104 τ ) be the autocorrelation function of a stationary stochastic signal?
No, as it doesn’t reach its peak in the origin.
No, as it is not even
No, as it takes negative values
No, as the value of the function at infinity is negative.
Yes.
No, as its Fourier transform is not even.
No, as its Fourier transform takes negative values.
No, as its Fourier transform is complex-valued.
May the functiun q(ω) = 20 sinc(20ω) be the power spectral density of a stochastic signal?
Yes, because the function is even
Yes, because the function is even and positive
Yes, because the function is even, real-valued and positive.
No, because the function may take negative values
No, because the function is odd.
No, because the function does not admit an inverse Fourier transform.
No, because the function is odd, and may take negative values.
Yes, because the function is the Fourier transform of an even function.
Random variables ξ and η are such that wξη(x, y) 6= wξ(x)wη(y). What can be said about the degree of correlation between them?(2 points)
They are not independent therefore they are correlated.
They are uncorrelated, as they are not independent
Nothing can be said about the degree of correlation between them
They are independent therefore they are uncorrelated
They are independent therefore they are correlated
The cloud of points (ξ, η) has a linear shape therefore they are correlated.
The cloud of points (ξ, η) has a linear shape therefore they are uncorrelated
The degree of correlation is very high.
What can be said about the ergodicity in the sense of the mean of a signal the autocorrelation function of which is Rξ(τ ) = 2 cos(2π103 τ )?
The signal is ergodic in the sense of the mean
The signal is not ergodic in the sense of the mean, as it is not stationary.
The signal is ergodic in the sense of the mean, because the autocorrelation function depends only on t1 − t2.
The signal is ergodic in the sense of the mean, because it is stationary.
Nothing can be said about the signal’s ergodicity in the sense of the mean.
The signal is ergodic in the sense of the mean, because it is ergodic in the sense of the autocorrelation.
The signal is not ergodic in the sense of the mean, because it is not ergodic in the sense of the autocorrelation.
The signal is not ergodic in the sense of the mean, because its autocorrelation function is periodic.
May function Rξ(τ ) = sinc(20πτ ) cos(2π104 τ ) be the autocorrelation function of a stationary stochastic signal?
No, as it doesn’t reach its peak in the origin.
No, as it is not even.
No, as it takes negative values.
No, as the value of the function at infinity is negative.
Yes.
No, as its Fourier transform is not even.
No, as its Fourier transform takes negative values.
No, as its Fourier transform is complex-valued.
What can be said on the joint probability density function (PDF) of a pair of independent random variables that have, each, a normal distribution of order one?
The joint PDF is never normal.
The joint PDF is not always normal.
The joint PDF is normal only if the variables are uncorrelated.
The joint PDF is normal only if the variables are correlated.
The joint PDF is always normal.
The joint PDF is normal only if the variables have zero mean.
The joint PDF is normal only if the variables’ PDFs of order one are identical.
Nothing can be said of the joint PDF.
Let ξ and η be two random variables having the covariance equal to - 0.5. Is it possible to compute the marginal probability density functions of the two random variables starting from the joint probability density function of order two?
Only if we knew the correlation coefficient between them
No, as we cannot tell whether they are independent.
Yes.
No, as we cannot tell how strongly they are correlated.
Yes, as they are correlated.(f) Yes, as they are independent.
Only if the joint probability density function was a Gaussian.
Only if they were independent.
What can be said about the ergodicity in the sense of the mean of stochastic signal ξ(t), given that ξ(t) = 0 and σξ(t) = 2 cos2 (t)?
The signal is ergodic, because it is stationary.
The signal is stationary, but it might or might not be ergodic.
We cannot assess ergodicity, as we do not know the signal’s autocorrelation function.
The signal is ergodic, even though it may not be stationary.
The signal is not ergodic, because it is not stationary.
We cannot assess stationarity, as we do not know the signal’s autocorrelation function.
The signal is stationary, but not ergodic.
In order to assess ergodicity, we should know the signal’s autocovariance function.
If the correlation between ξ and η is 0, then:
They are independent.
They are uncorrelated.
They are not independent
They are not uncorrelation
Nothing can be said about their degree of correlation
They are independent, but correlated
They are uncorrelated, nothing can be said about their dependence
Is stochastic signal with autocovariance function given by Kξ(τ ) = 10−|τ | 10 if |x| ≤ 10 0 otherwise ergodic in the sense of the mean?
No, as the function does not meet the condition of the ergodicity mean theorem
Yes, as the function is even
Yes, as the function has finite support therefore meets the condition of the ergodicity mean theorem.
It cannot be specified.
Yes, as the signal is stationary therefore ergodic in the sense of the mean.
No, as the signal mean is time-dependent.
Yes, as the function value as infinity tends to 0.
Yes, as the function is even and positive
If autocorrelation functions of both input and output signals of a linear, time-invariant system are known, can the system’s point-spread function be determined?
(a) Yes, always.
Only if we additionally know the intercorrelation between the two signals.
Not always, as the output signal’s power spectral density may have zeroes.
Only if the system’s transfer function is positive.
No, as we cannot always compute the power spectral densities of the two signals.
Not always, as the input signal’s power spectral density may have zeroes.
No, as we additionnally need the two means.
No, as we cannot compute the transfer function’s phas
What can be said of two random variables, knowing that the mean of their product equals the product of their means?
They are independent, but nothing can be said of the correlation between them.
Nothing, the mean of the product always equals the product of the means.
They are dependent, but uncorrelated.
They are uncorrelated, but nothing can be said of the dependence between them.(e) They are independent therefore uncorrelated.
Nothing, we additionnally need the covariance between the two variables.
They are correlated therefore dependent.
They are independent, to compute the correlation coefficient we need the two standard deviations.
Let ξ and η be two uncorrelated random variables having a Gaussian distribution (of order one). Is it possible to compute the joint probability density function of both ξ and η?
Nu, as they are not independent.
Yes, as they are independent.
Da, as two uncorrelated Gaussian random varaiables are independent too.
No, as two uncorrelated Gaussian random varaiables are independent too.
Yes, as they are uncorrelated.
No, as they are uncorrelated
No, as the correlation coefficient is unknown.
What can be said about the ergodicity in the sense of the mean of stochastic signal ξ(t), given that ξ(t) = 0 and σξ(t) = 2 cos2 (t)?
The signal is ergodic, because it is stationary.
The signal is stationary, but it might or might not be ergodic.
We cannot assess ergodicity, as we do not know the signal’s autocorrelation function.
The signal is ergodic, even though it may not be stationary.
The signal is not ergodic, because it is not stationary.
We cannot assess stationarity, as we do not know the signal’s autocorrelation function.
The signal is stationary, but not ergodic.
In order to assess ergodicity, we should know the signal’s autocovariance function.
If the correlation between ξ and η is 0, then:
They are independent.
They are uncorrelated.
They are not independent.
They are not uncorrelation.
Nothing can be said about their degree of correlation.
They are independent, but correlated.
They are uncorrelated, nothing can be said about their dependence.
They are both independent and uncorrelated
May two mutually exclusive events be independent too?
Always.
Never.
Only when one of them is the certain event.
Only when one of them is the impossible event.
Only when one of them includes the other one.
Only when the two events are complementary
Only when events are uncorrelated.
Only when events are correlated.
What can be said of the stationarity of a stochastic signal which is ergodic in the sense of the mean?
The signal may by stationary.
The signal may is wide-sense stationary, not strict-sense stationary.
The signal may is strict-sense stationary, not wide-sense stationary.
The signal is wide-sense stationary.
If the signal were ergodic in the sense of autocorrelation, then it would be stationary.
The signal is not stationary.
The signal is stationary only in the sense of the mean, not in the sense of autocorrelation.
Nothing can be said on signal’s stationarity.
Let ξ and η be two random variables having the covariance equal to - 0.5. Is it possible to compute the marginal probability density functions of the two random variables starting from the joint probability density function of order two?
Only if we knew the correlation coefficient between them.
No, as we cannot tell whether they are independent.
Yes.
No, as we cannot tell how strongly they are correlated.
Yes, as they are correlated.
Yes, as they are independent.
Only if the joint probability density function was a Gaussian.
Only if they were independent.
What is the significance of the fact that the cumulative distribution function of a random variable is constant over an interval [a, b]?
The random variable is discrete.
The probability density function of the random variable will contain a Dirac impulse of area (b − a)
The probability that the random variable takes values inside the [a, b] interval is constant too.
There is a high probability that the random variable takes values inside the [a, b] interval.
There is a low probability that the random variable takes values inside the [a, b] interval.
The random variable cannot take values inside the [a, b] interval.
The probability density function will be discontinuous at points a and b.
The random variable cannot take values outside the [a, b] interval.
What can be said of the stationarity of a stochastic signal the power spectral density of which is qξ(ω) = exp (−4/100+ω2)
It cannot be stationary, as its power spectral density is even.
Nothing can be said of its stationarity, as we don’t know if the signal’s autocorrelation function depends only on one argument.
One should compute its autocorrelation function in order to assess stationarity.
It cannot be stationary, as its power spectral density is not complex-valued.
Nothing can be said, as we don’t know if the signal’s mean is time-independent
The signal is definitely stationary.
The function qξ above cannot be the power spectral density of a signal, as it does not meet all the properties.
It cannot be stationary, as its power spectral density is real-valued.
If autocorrelation functions of both input and output signals of a linear, time-invariant system are known, can the system’s point-spread function be determined?
Yes, always.
Only if we additionally know the intercorrelation between the two signals.
Not always, as the output signal’s power spectral density may have zeroes.
Only if the system’s transfer function is positive.
No, as we cannot always compute the power spectral densities of the two signals.
Not always, as the input signal’s power spectral density may have zeroes.
No, as we additionnally need the two means.
No, as we cannot compute the transfer function’s phase.
What is the significance of a discontinuity in the cumulative distribution function of a random variable? (
The random variable is discrete.
The probability density function of the random variable will be discontinuous too.
The random variable cannot take the value where the discontinuity occurs.
The probability density function of the random variable will contain a train of Dirac impulse
The random variable is continuous.
The random variable will have non–zero probability to take the value where the discontinuity occurs.
The random variable is mixed.
The probability that the random variable takes values in a small interval around the discontinuity is zero.
What can be said of two random variables, knowing that the mean of their sum equals the sum of their means?
They are independent, but nothing can be said of the correlation between them.
Nothing, the sum of the product always equals the product of the sums
They are dependent, but uncorrelated.
They are uncorrelated, but nothing can be said of the dependence between them.
They are independent therefore uncorrelated
In order to assess their independence, we furthermore need the covariance between the two variables.
They are correlated therefore dependent.
They are independent, to compute the correlation coefficient we need the two standard deviations.
The correlation between random variables ξ and η that have a joint normal distribution, with parameters m1 = 4, m2 = 2, σ1 = 4, σ2 = 3, ϝ = −0, 5 is:
0
1
2
3
What can be said of two random variables, knowing that the mean of their product equals the product of their means?
They are independent, but nothing can be said of the correlation between them.
Nothing, the mean of the product always equals the product of the means.
They are dependent, but uncorrelated.
They are uncorrelated, but nothing can be said of the dependence between them.
They are independent therefore uncorrelated.
Nothing, we additionnally need the covariance between the two variables.
They are correlated therefore dependent.
They are independent, to compute the correlation coefficient we need the two standard deviations.
What can be said of two random variables, knowing that the mean of their sum equals the sum of their means?
They are independent, but nothing can be said of the correlation between the
Nothing, the sum of the product always equals the product of the sums.
They are dependent, but uncorrelated.
They are uncorrelated, but nothing can be said of the dependence between them.
They are independent therefore uncorrelated.
In order to assess their independence, we furthermore need the covariance between the two variables.
They are correlated therefore dependent.
They are independent, to compute the correlation coefficient we need the two standard deviations.
What can be said on the joint probability density function (PDF) of a pair of independent random variables that have, each, a normal distribution of order one?
The joint PDF is never normal.
The joint PDF is not always normal.
The joint PDF is normal only if the variables are uncorrelated.
The joint PDF is normal only if the variables are correlated
The joint PDF is always normal.
The joint PDF is normal only if the variables have zero mean.
The joint PDF is normal only if the variables’ PDFs of order one are identical.
Nothing can be said of the joint PDF.
What can be said about the ergodicity in the sense of the mean of a signal the autocorrelation function of which is Rξ(τ ) = 2 cos(2π103 τ )
The signal is ergodic in the sense of the mean.
The signal is not ergodic in the sense of the mean, as it is not stationary.
The signal is ergodic in the sense of the mean, because the autocorrelation function depends only on t1 − t2.
The signal is ergodic in the sense of the mean, because it is stationary.
Nothing can be said about the signal’s ergodicity in the sense of the mean.
The signal is ergodic in the sense of the mean, because it is ergodic in the sense of the autocorrelation
The signal is not ergodic in the sense of the mean, because it is not ergodic in the sense
The signal is not ergodic in the sense of the mean, because its autocorrelation function is
Is stochastic signal with autocovariance function given by Kξ(τ ) = 10−|τ | 10 if |x| ≤ 10 0 otherwise ergodic in the sense of the mean?
No, as the function does not meet the condition of the ergodicity mean theorem.
Yes, as the function is even.
Yes, as the function has finite support therefore meets the condition of the ergodicity mean theorem.
It cannot be specified.
Yes, as the signal is stationary therefore ergodic in the sense of the mean.
No, as the signal mean is time-dependent.
Yes, as the function value as infinity tends to 0.
Yes, as the function is even and positive.
What is the significance of the fact that the cumulative distribution function of a random variable is constant over an interval [a, b]?
He random variable is discrete.
The probability density function of the random variable will contain a Dirac impulse of area (b − a).
The probability that the random variable takes values inside the [a, b] interval is constant too.
There is a high probability that the random variable takes values inside the [a, b] interval.
There is a low probability that the random variable takes values inside the [a, b] interval.
The random variable cannot take values inside the [a, b] interval.
The probability density function will be discontinuous at points a and b
The random variable cannot take values outside the [a, b] interval.
What can be said of two random variables that have a correlation coefficient equal to -0.9?
They are poorly correlated, as the correlation coefficient is negative
They are poorly correlated, as the correlation coefficient has a very low absolute value
In order to assess the degree of correlation between them, we further need their variances
They are highly correlated, as the correlation coefficient has a very high absolute value
It is not possible for correlation coefficient to have negative values.
In order to assess the degree of correlation between them, we further need their means and variances.
They are poorly correlated, as the correlation coefficient has a very low value.
The linear dependence between them is quite poor.
What can be said of two random variables that have a correlation coefficient equal to 6?
Sunt independente.
Sunt decorelate.
Nu sunt independente.
Nu sunt decorelate.
Nu se poate spune nimic despre gradul lor de corelat¸ie.
Sunt independente, dar corelate.
Sunt decorelate, dar nu se poate spune nimic despre gradul lor de dependent¸˘a.
Sunt ¸si independente, ¸si decorelate.
Is stochastic signal with autocovariance function given by Kξ(τ ) = 10−|τ | 10 if |x| ≤ 10 0 otherwise ergodic in the sense of the mean?
Nu, intrucˆat funct¸ia nu respect˘a teorema ergodicit˘at¸ii medie
Yes, as the function has finite support therefore meets the condition of the ergodicity mean theorem.
Da, c˘aci funct¸ia este par˘a.
Da, c˘aci funct¸ia, avˆand suport finit, respect˘a teorema ergodicit˘at¸ii mediei.
Nu se poate preciza cu sigurant¸a.
Da, c˘aci semnalul este stat¸ionar, ¸si deci, implicit ergodic ˆın sensul mediei.
Nu, ˆıntrucˆat media semnalului depinde de timp.
Da, c˘aci valoarea la infinit a funct¸iei tinde la 0.
Da, c˘aci funct¸ia este par˘a ¸si pozitiv˘a.
Random variables ξ and η are such that wξη(x, y) 6= wξ(x)wη(y). What can be said about the degree of correlation between them?(2 points)
Nu sunt independente, deci nici decorelate.
Sunt decorelate, pentru ca nu sunt independente.
Nu se poate spune nimic despre gradul de corelat¸ie dintre ele.
Sunt independente, deci decorelate.
Sunt independente, deci corelate.
Norul de puncte (ξ, η) este liniar, deci sunt corelate.
Norul de puncte (ξ, η) este liniar, deci sunt decorelate.
Gradul de corelatie este foarte ridicat.
What can be said of the stationarity of a stochastic signal which is ergodic in the sense of the mean?
Semnalul poate fi, sau nu, stat¸ionar.
Semnalul este stat¸ionar ˆın sens larg, dar nu ˆın sens strict.
Semnalul este stat¸ionar ˆın sens strict, dar nu ˆın sens larg
Semnalul este stat¸ionar ˆın sens larg.
Dac˘a semnalul ar fi ¸si ergodic ˆın sensul autocorelat¸iei, atunci ar fi stat¸ionar.
Semnalul nu este stat¸ionar.
Semnalul este stat¸ionar numai ˆın sensul mediei, nu ¸si ˆın sensul autocorelat¸iei.
Nu se poate spune nimic.
Corelat¸ia dintre variabilele aleatoare independente ξ ¸si η, avˆand, ambele, o distribut¸ie uniform˘a pe intervalul [0, 2] este:
0
1
0.5
0.25
0.75
0.2
0..6
0.8
If autocorrelation functions of both input and output signals of a linear, time-invariant system are known, can the system’s point-spread function be determined?
Da, ˆıntotdeauna.
Numai ˆın situat¸ia ˆın care ¸stim ¸si intercorelat¸ia dintre cele dou˘a semnale.
Nu ˆıntotdeauna, c˘aci densitatea spectral˘a de putere a semnalului de ie¸sire poate avea zerouri.
Numai ˆın cazul ˆın care funct¸ia de transfer a sistemului are valori pozitive.
) Nu, ˆıntrucˆat nu putem calcula ˆıntotdeauna densit˘at¸ile spectrale de putere ale celor dou˘a
Nu ˆıntotdeauna, c˘aci densitatea spectral˘a de putere a semnalului de intrare poate avea
Nu, ˆıntrucˆat avem nevoie ¸si de mediile celor dou˘a semnale.
Nu, ˆıntrucˆat nu putem calcula faza funct¸iei de transfer.
Evenimentele A ¸si B sunt astfel ˆıncˆat P(A|B) = 0. Ce se poate spune despre independent¸a dintre A ¸si B?
A ¸si B sunt independente.
A ¸si B sunt dependente, dar decorelate.
A ¸si B sunt independente doar dac˘a unul dintre ele este evenimentul imposibil.
A ¸si B sunt dependente.
A ¸si B sunt independente doar dac˘a unul dintre ele ˆıl include pe cel˘alalt.
Nu se poate spune nimic despre independent¸a lui A ¸si B.
A ¸si B sunt corelate, dar independente.
A ¸si B sunt dependente doar dac˘a unul dintre ele ˆıl include pe cel˘alalt.
May the functiun q(ω) = 20 |sinc(20ω)| be the power spectral density of a stochastic signal?
Da, pentru c˘a este o funct¸ie par˘a.
Da, pentru c˘a este o funct¸ie par˘a ¸si real˘a.
Da, pentru c˘a este o funct¸ie par˘a ¸si real˘a ¸si pozitiv˘a.
Nu, pentru c˘a este o funct¸ie care poate lua valori negative.
Nu, pentru c˘a este o funct¸ie impar˘a.
Nu, ˆıntrucˆat nu admite transformat˘a Fourier invers˘a.
Nu, pentru c˘a este o functˆıe impar˘a, ce poate lua valori negative.
Da, pentru c˘a este transformata Fourier a unei funct¸ii pare.
{"name":"Depi", "url":"https://www.quiz-maker.com/QPREVIEW","txt":"Test your knowledge on stochastic processes and probability theory with our comprehensive quiz. With 56 challenging questions, this quiz covers a range of topics including random variables, autocorrelation, and independence.Understand the intricacies of stochastic signals.Evaluate your grasp of probability density functions.Explore the relationships between random variables.","img":"https:/images/course8.png"}
More Quizzes
Experimental Design and Data Analysis Quiz 1
311624
Statistics Confidence Quiz
1890
Quiz 3
5219
What bread are you?
6332157
CLASSIFICATION AND OPERATION ON SIGNAL AND SYATEMS
221129
Data Literacy Post Test 21 April 2023
1050
MATLAB HandsOn Workshop
12629
Spectrum analyzer Theory quiz
10512
Six Sigma Yellow Belt
5240
Session 1
4228
DV007
10525
Data Analytics Pretest
10512